Why Is Deep Learning Challenging for Printed Circuit Board (PCB) Component Recognition and How Can We Address It?
a) it is an original missing hole defect in the image; (b) random crop... | Download Scientific Diagram
![PDF] FICS-PCB: A Multi-Modal Image Dataset for Automated Printed Circuit Board Visual Inspection | Semantic Scholar PDF] FICS-PCB: A Multi-Modal Image Dataset for Automated Printed Circuit Board Visual Inspection | Semantic Scholar](https://d3i71xaburhd42.cloudfront.net/de274e9ba67a09e80816b98818dcd2debe10350d/1-Figure1-1.png)
PDF] FICS-PCB: A Multi-Modal Image Dataset for Automated Printed Circuit Board Visual Inspection | Semantic Scholar
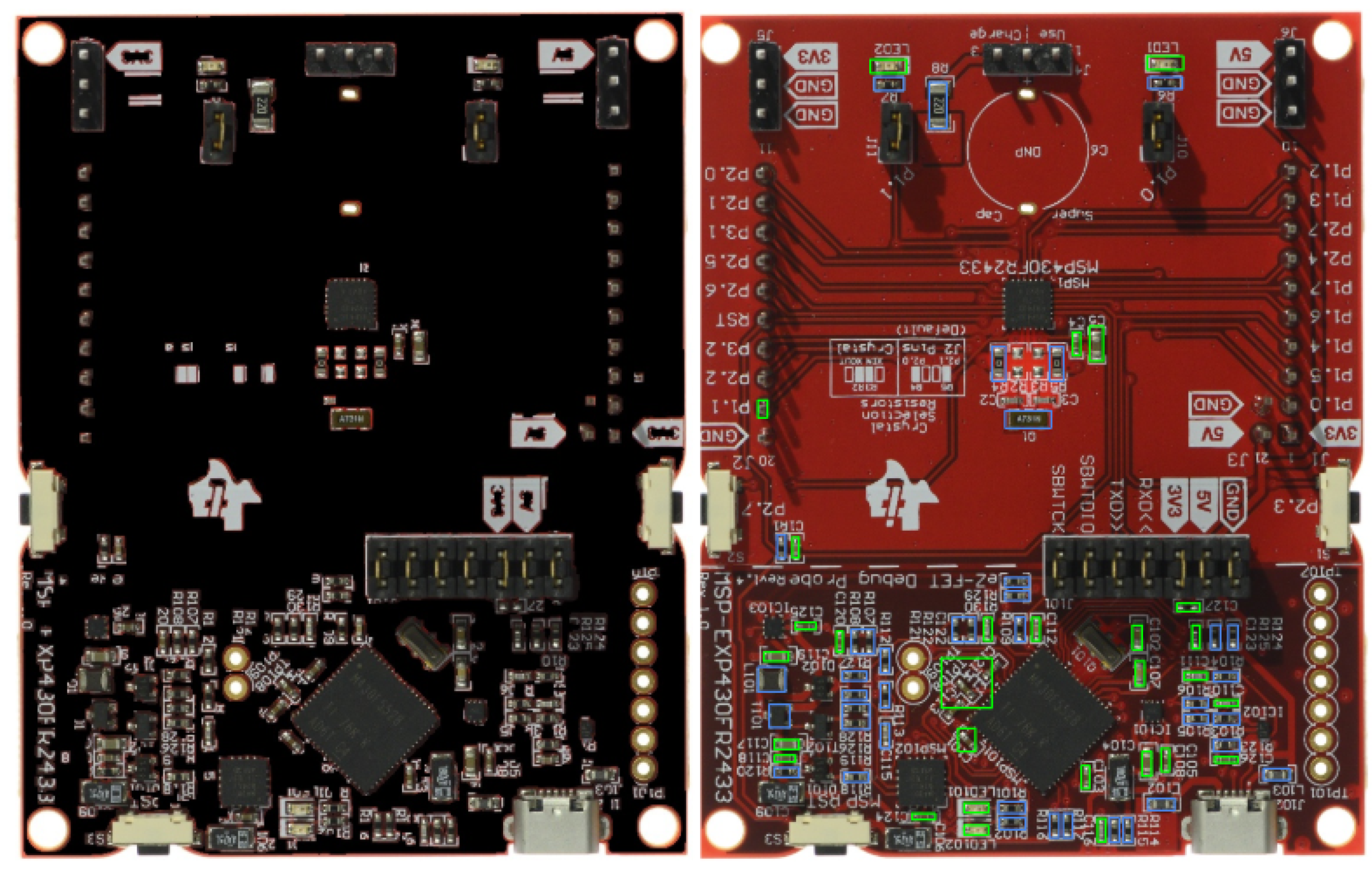
Cryptography | Free Full-Text | Why Is Deep Learning Challenging for Printed Circuit Board (PCB) Component Recognition and How Can We Address It? | HTML

Strongest feature points of the detected missing component based on... | Download Scientific Diagram
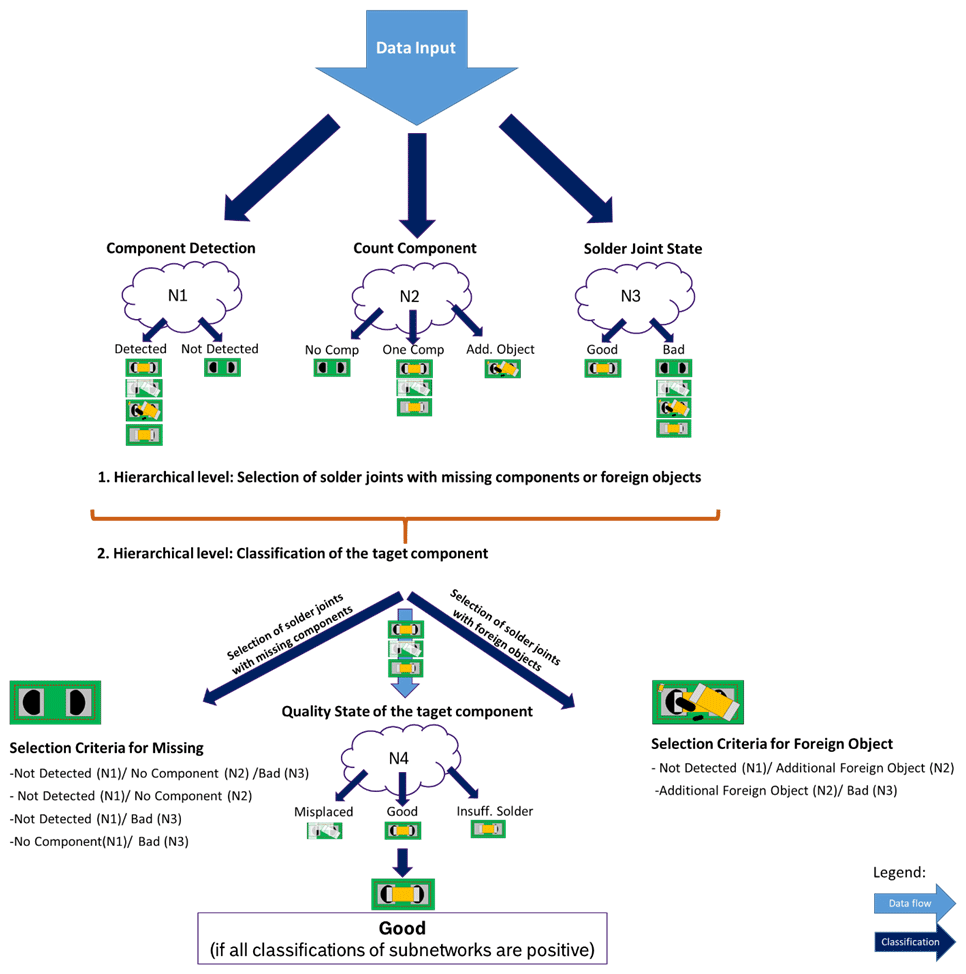
JSSS - Intelligent fault detection of electrical assemblies using hierarchical convolutional networks for supporting automatic optical inspection systems
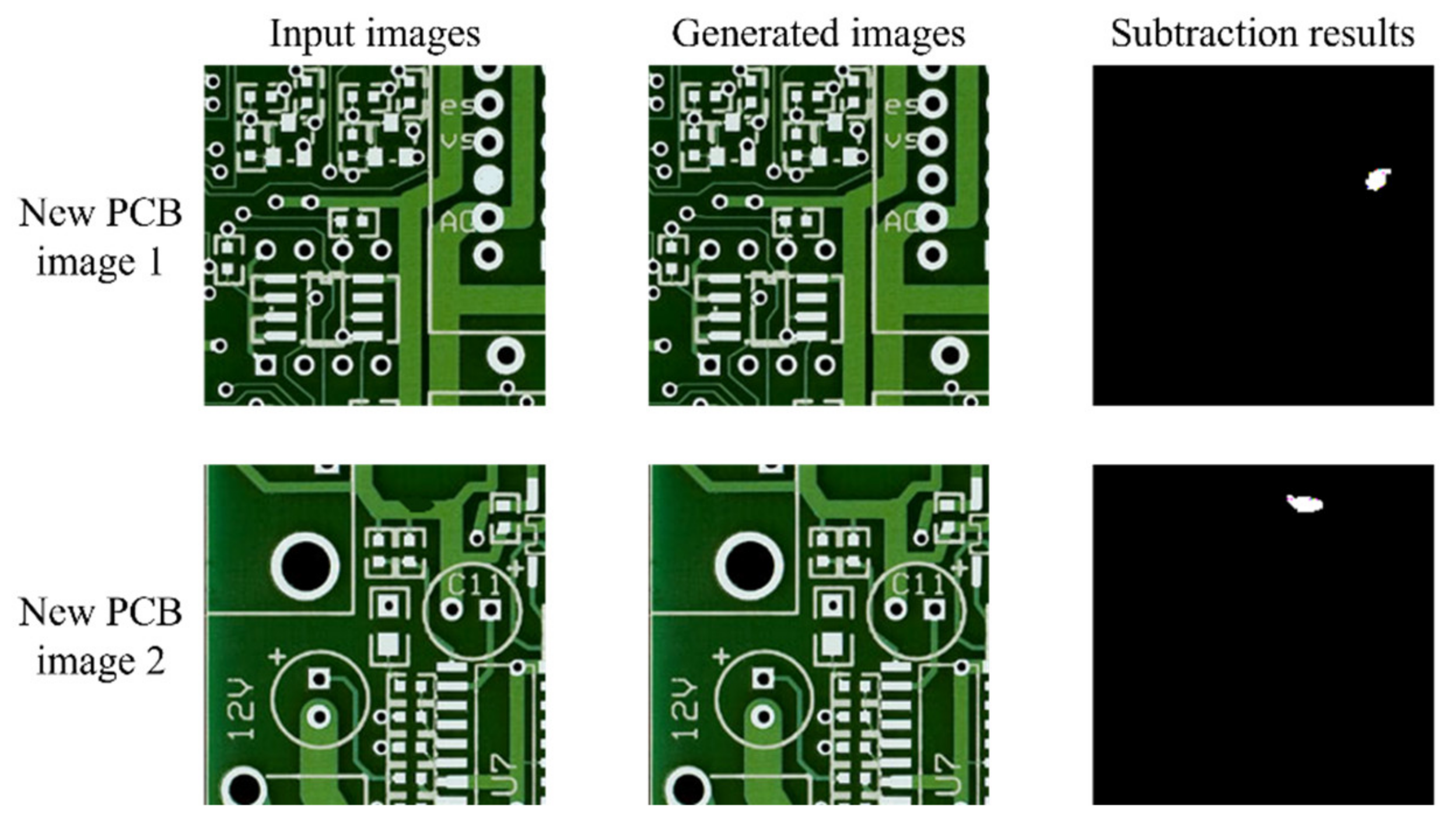
Sensors | Free Full-Text | Printed Circuit Board Defect Detection Using Deep Learning via A Skip-Connected Convolutional Autoencoder | HTML
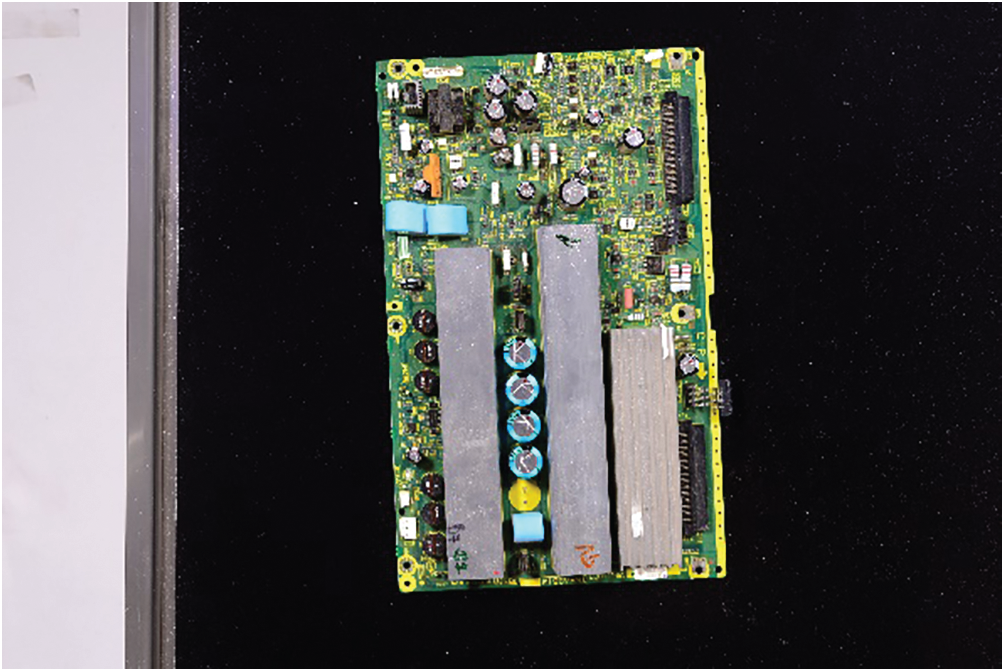